AI Impacting Industry
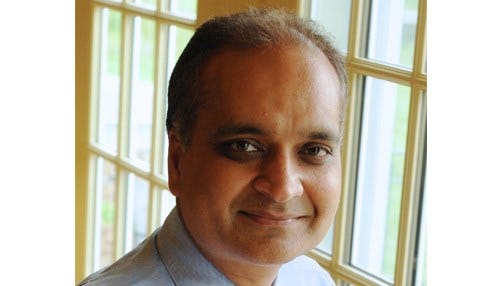
Subscriber Benefit
As a subscriber you can listen to articles at work, in the car, or while you work out. Subscribe NowArtificial Intelligence is improving and impacting industries, businesses and governments worldwide. For business leaders new to AI, there’s probably uncertainty about its ability to help their organization. Here are a few specific use cases that show the impact.
Business professionals are talking about and beginning to implement Artificial Intelligence into their work place. Here are four great examples showing how AI benefits industry.
FINANCIAL: FRAUD PREVENTION
The convenience of credit cards, debit cards and smartphones creates irresistible targets for criminals. Mechanical countermeasures like holographic images and two-factor authentication present, at best, an inconvenience to determined fraudsters. They’ve figured out how to work around them.
AI algorithms use one of the most reliable defenses against financial fraud — the customer’s own behavior. Every financial transaction generates valuable digital data. Fraud-fighting algorithms correlate this data, scan for useful patterns and weigh the likelihood that the legitimate owner of a debit card, for instance, is the one making the transaction.
Let’s say a person uses their credit card during a business trip to Milwaukee. When a credit card is used there, an AI algorithm knows it’s really you because the credit card also was used to buy the airline ticket to Milwaukee.
AI algorithms scan dozens or hundreds of transactions over previous months and years. These scans find patterns to establish the legitimate customer’s behavioral signature. As the learning algorithms teach themselves to model users’ behavior more accurately, they get better at tackling anomalies that might produce a false positive. That means less aggravation for customers who don’t have to call in to register travel and more roadblocks for crooks who would work outside the customer’s behavior.
AUTOMOTIVE: PREDICTIVE MAINTENANCE
There are predictions that bots will do all the driving someday. But for now, people who own cars and trucks have to sort out a bunch of everyday automotive maintenance chores manually. Today’s cars can count the miles on the odometer and deliver an alert when it’s time to change the oil. Scheduling an oil change means playing phone tag with the dealer and syncing your calendar with the dealer’s availability. There’s a better way, thanks to AI.
An AI engine can scan driving patterns and predict when your change-the-oil light will come on. Another AI engine scans availability in the dealer’s garage. The AI system knows you’ve gone to the dealer on Tuesdays the last five times.
All these variables trigger a message telling you that your oil-change light is about to come on. You’ll be asked which day you’d like to get the oil change and which dealer you’d like to use. It’s conversational in the sense that you can talk to a mobile app and complete the transaction hands-free while you’re driving.
The concept applies across the automotive ecosystem. The best part, predictive maintenance will drive cost savings and production efficiency for OEMs and their vendors.
PHARMACEUTICALS: OPTIMIZING CLINICAL TRIALS
Healthcare is a natural fit for AI data modeling because medicine also is a scientific discipline built on empirical data. Scientific studies generate massive volumes of data that can be vetted by peer review, decreasing the likelihood of errors. AI engines can be trained to correlate and analyze the results of multiple studies to find patterns revealing insights that humans would probably miss.
Developing new drugs starts in the laboratory and eventually moves to clinical trials that establish whether the medication works as expected. The process can take five to 12 years and cost billions of dollars.
Occasionally, a medication succeeds in laboratory tests on animals but fails in clinical testing on humans. Clinical trial failures equate to hundreds of millions of dollars wasted on scientific dead ends. When news of trial failures hits the financial press, investors may lose confidence and company stock prices may take a beating. Thus, pharmaceutical manufacturers have a strong incentive to identify flaws in their studies, limit losses and prevent failure.
With an AI-enabled decision matrix that asks if a trial will fail or succeed companies can mitigate risk. The matrix takes the emotions out of a hard decision. It looks at all the data to help decide whether to stop a clinical trial when AI is saying it’s not going to succeed.
GOVERNMENT: WORKER SAFETY AND PRODUCTIVITY
More than four million people are employed by U.S. government. A workforce this large generates two fundamental challenges: safety and productivity. AI and machine learning algorithms are helping the government on both counts.
Many other U.S. employees face potentially fatal risks. For instance, the U.S. Mine Safety and Health Administration (MSHA) sends inspectors deep into coal mines to probe for problems that could produce catastrophic accidents.
DMI partnered with MSHA to pioneer an inspection platform that made inspectors more efficient. Instead of carrying laptop computers, digital cameras and thick regulation books down into the mines, the inspectors combined all these tools in a lightweight tablet computer. These tools shrank inspection cycles from months to days.
AI algorithms analyze inspection data and correlate it with data from other mines and inspections. AI gives the ability to predict an accident in a specific mine based on its equipment, conditions, workforce availability, inspection data and more. Risks can be identified and fixed to ensure worker safety.
Using real-time data to prevent accidents is a game changer in industries the government regulates. Moreover, predictive capability also helps the military maintain its arsenal of complex machinery.
Niraj Patel is senior vice president of artificial intelligence for DMI with 21 global offices including Indianapolis. DMI helps clients navigate the technology revolution through a human-centric, mobile-first approach. DMI is continuously recognized by Gartner and Forrester as a leader in mobile IT services.